In the #3 installment of the Neuroergonomics Conference webinar series, Fabien Lotte (National Institute for Research in Computer Science and Control, France) and Stephen Fairclough (Liverpool John Moores University, UK) will moderate a special series of talks that explain the domain of “Neurotechnology and Systems Neuroergonomics”.
Date: 3 March 2021
Subscribe for reminders
Participation Details
This webinar will be broadcasted live over our YouTube channel. To interact with fellow participants, please join our Discord server.
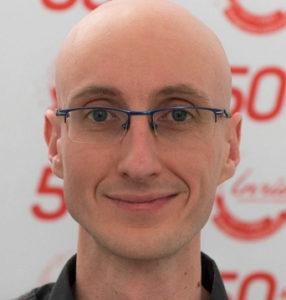
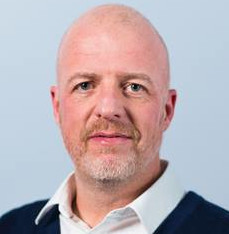
Time
Program
09.30 (EDT)
15.30(CET)
22.30(CST)
Meet & Greet
Meet up on our Discord server.. Make new friends catch up with old ones.
10.00 (EDT)
16.00(CET)
23.00(CST)
Welcome remarks
Fabien Lotte (National Institute for Research in Computer Science and Control, France)
Stephen Fairclough (Liverpool John Moores University, UK)
10.15 (EDT)
16.15 (CET)
23.15 (CST)
Towards Neuroadaptive Technology based on cognitive probing
Thorsten Zander (Brandenburg Technical University, Germany)
10.45 (EDT)
16.45 (CET)
23.45 (CST)
EEG-based passive Brain-Computer Interfaces in operational environments: from laboratory evidences to real scenarios
Gianluca Di Flumeri (Sapienza University of Rome, Italy)
11.15 (EDT)
17.15 (CET)
00.15 (CST)
Implicit brain-machine interactions in navigation and target identification tasks
Mahnaz Arvaneh (University of Sheffield, UK)
11.45 (EDT)
17.45 (CET)
00.45 (CST)
Using physiological synchronization and hyperscanning to enhance pair and group interaction
Domen Novak (University of Wyoming, USA)
Towards Neuroadaptive Technology based on cognitive probing
A user’s interaction with technology can be improved by integrating an implicit information flow from their brain to the machine, based on Passive Brain-Computer Interfaces. The resulting Neuroadaptive Technology optimizes its own state and actions according to changes in the communicated aspects of its users cognitive and affective state, to support the ongoing interaction.
Neuroadaptation can be based on a task-specific user model that collates mental responses of the user to the associated context. One tool to maintain and refine such a user model during an ongoing interaction is so-called cognitive probing.
In the first part of this talk a framework for cognitive probing will be presented, followed by a brief summary of an exemplary study that is using this tool.
EEG-based passive Brain-Computer Interfaces in operational environments: from laboratory evidences to real scenarios
BCI technology has rapidly and considerably advanced in formulation and aims. In the last decade, the BCI field went through a gigantic revolution: its concept evolved from the “overt” detection of human intention to the “covert” assessment of human mental states. Such a concept would imply enormous benefits in safety-relevant operational environments where it is paramount to ensure that the operator always works at the best of its psychophysiological conditions.
The talk will present the intriguing results of two recent studies based on the application of EEG-based passive BCI in close-to-real scenarios, and then it will discuss what is still missing, despite the large evidence coming from scientific literature, to deploy this technology in real scenarios.
Implicit brain-machine interactions in navigation and target identification tasks
There is a performance bottleneck in many BCIs, as users are required to control each low-level action in order to achieve a high-level goal. For example, users may need to consciously generate brain signals to move a cursor, prosthesis, or assistive robot, step-by-step to a desired location. This places a high mental workload on the user. Recent studies have shown the possibility of using “cognitive probing” to reduce the mental burden of current BCIs.
This is achieved by monitoring brain signals generated spontaneously while users merely observe the machine’s actions, and using these signals as feedback to help the machine perform the desired task. These studies have mostly been based on distinguishing correct actions from erroneous ones, by detecting error-related potentials.
In this talk we will discuss the possibility of obtaining more detailed information from passive brain signals than simply whether an action was correct or erroneous. Building on this, we will show that it is possible to sub-classify different types of navigational errors against each other, and to sub-classify different correct navigational actions against each other. Bringing these advances together, we will present the foundation of a new frame-work for detailed implicit communication between brain and machine, by embedding the real-time EEG classification outputs in a dynamic probabilistic model of the most likely target loci based on the previous actions of the robot. This facilitates semi-autonomous robot control, through a more efficient and user-friendly brain-machine interaction.
Using physiological synchronization and hyperscanning to enhance pair and group interaction
Physiological synchronization is a phenomenon in which the central and autonomic nervous system responses of two or more people gradually converge as the individuals work together, talk to each other, or simply experience things together. This synchronization can be measured using many sensors; if using brain measurements, the process is called hyperscanning.
The degree of synchronization provides rich information about social functioning and relationships: for example, collaboration quality, degree of rapport, and degree of shared engagement. Thus, it could be used to enhance pair or group scenarios – for example, by dynamically adapting a task in order to maximize synchronization or by providing visual feedback about the synchronization level to participants. This talk will present three representative examples of research on physiological synchronization: in competition, communication, and group movie watching.